The Importance of Data Governance and Data Quality for Digital Transformation
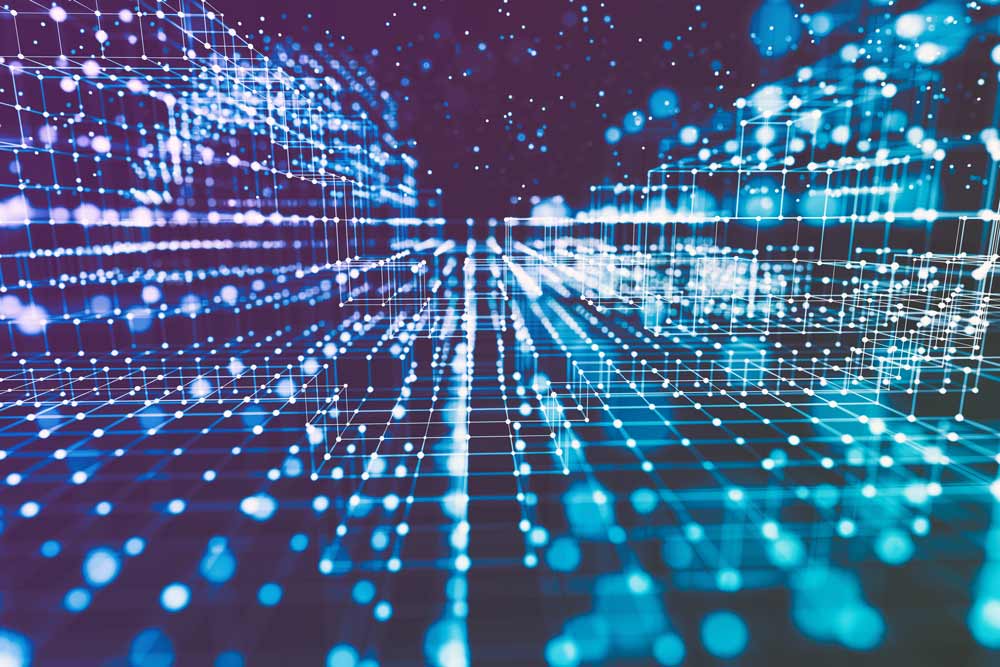
More and more companies are becoming aware of the positive impact that supporting their operational decisions with a consistent and cross-cutting data policy can have on their activity.
The process of adopting a data-driven strategy, however, is not usually quick, nor, in the vast majority of cases, is it easy. Once an organisation has overcome the usual resistance inherent in any major paradigm shift, it is essential to make the objectives to be achieved visible and know how to effectively manage the speed and direction set. This can only be achieved through strict control and mentoring throughout the different stages that make up the digital transformation process.
First, the organisation realises that it possesses and continuously generates new data that it could use to operate more efficiently (data stage). Then it’s time to identify the first use cases, those that are most obvious and straightforward to address, and begin to use data in production (analysis stage). The next step is to understand the benefits of this new way of making business decisions and to extend the operation to more and more processes (insight stage). Finally, when the organisation not only makes every decision based on an intelligent use of the available data, but when every member of the organisation takes ownership of this culture and acts accordingly, we reach the strategic stage. Becoming a data-driven organisation therefore requires obtaining the necessary levers to unlock the value of the data the organisation generates.
Data governance and data quality
Dos pilares esenciales en la consecución y sostenimiento del status que caracteriza a las organizaciones data-driven son los llamados data governance y data quality. Con frecuencia vemos que estos conceptos se mezclan y usan indistintamente, incluso hasta el punto de sugerir una suerte de jerarquía entre ellos. Por ejemplo, en ocasiones se plantea ‘data quality’ como un mero componente de ‘data governance’. No obstante, suTwo essential pillars in achieving and sustaining the status that characterises data-driven organisations are data governance and data quality. We often see these concepts mixed and used interchangeably, even to the point of suggesting a kind of hierarchy between them. For example, ‘data quality’ is sometimes approached as a mere component of ‘data governance’. However, their roles and responsibilities differ greatly.
Data quality refers to ensuring that all data in the organisation is complete, accurate and ready for business managers to analyse, share and convert into actionable knowledge for decision making. While data quality has always been important, its strategic value has increased proportionally as organisations have generated increasing volumes of data from a variety of sources and formats.
Data governance, however, is about creating a framework and rules according to which organisations intend to use data throughout their life cycle. The primary role is to ensure that the relevant data serves each critical business function. In one sense, data governance focuses on the “what” and the “who”, while data quality focuses on the “how”.
Purpose and strategy
As can already be guessed, these are operations with complementary functions. Data quality has one basic purpose: to organise and cleanse data to ensure that it is complete, timely and accurate for its intended purpose (i.e. actionable data). Conversely, without quality data on which to build a framework, there is no reason to have a data governance process. In other words, data governance is meaningless without a constant supply of quality data.
A sound data quality strategy should include three main components:
- Be aligned at the organisational level with business rules
- Build in the right technology to ensure data quality
- Commit the necessary technical and personnel resources to maintain the defined quality standards over time
The following are examples of some of the most important data quality metrics that we can implement:
- Statistics on the number of data errors identified and/or corrected per unit of time (e.g. per week or per month)
- Accuracy and error rates in datasets or in data streams, with alerts if acceptable levels are exceeded
- Quantitative measures of completeness, consistency, integrity and timeliness
- Categorisation and estimation of the impact of data quality problems, as well as potential correction mechanisms
- Assessment of quality levels in data definition, metadata and data catalogues
- End-user feedback on perceived data quality
Institutional movements
Recently, the Data Office, under the Secretary of State for Digitalisation and Artificial Intelligence, has promoted and participated in the generation of the national specification UNE 0080:2023 called “Evaluation Guide for Data Governance, Management and Quality Management”, which responds to the need of both public and private organisations to have standardised mechanisms for the evaluation of their processes in terms of data governance, management and quality. The document is based, in turn, on the definition of processes contained in the specifications for Data Governance UNE 0077:2023, Data Management UNE 0078:2023, and Data Quality Management UNE 0079:2023.
This new specification is aimed at those responsible for implementing and monitoring data governance, data management and data quality management processes in their respective organisations, so as to ensure that they are functioning well or, if necessary, are able to implement or improve them, as well as at consultants and auditors who need to carry out an assessment of these processes.
Strengthening companies’ digitisation processes
From Gradiant we accompany the companies in our environment and help them to strengthen their digitisation processes with data orientation considering both axes: data governance and data quality.
On the one hand, we design and provide technologies to assess data quality by identifying problems and errors before they propagate to analytical processing and/or artificial intelligence applications.
On the other hand, we provide advice on the implementation of internal standards and data management policies, so that the processes of acquisition, storage, exploitation and disposal of data are properly defined, identifying the roles and levels of access appropriate to each scenario, in line with your business strategy.
Author: Rafael Martínez, Technical Manager of Big Data Analytics at Gradiant