Challenges and solutions of conventional AI in the healthcare sector
Artificial intelligence (AI) applied to healthcare has the potential to enhance diagnostic processes and improve the quality of medical care, with an estimated improvement in outcomes ranging from 30% to 40%. According to a report by Frost & Sullivan, AI can also reduce treatment costs by half. AI applications in healthcare are diverse, spanning early disease detection to managing vast patient data to personalize treatments, all while ensuring privacy and data protection. Advanced algorithms, such as those used in medical imaging analysis (e.g., CT scans and MRIs), have demonstrated precision comparable to human experts, accelerating diagnoses and reducing errors.
However, despite these advantages, medical diagnostic AI models face significant challenges, including potential biases in the data and the high sensitivity of such data, necessitating robust protection mechanisms. Biases may arise if the data used to train algorithms do not adequately represent all demographic groups, leading to inaccurate diagnoses. Additionally, handling sensitive patient information raises serious concerns about security and compliance with privacy regulations.
Reflecting Societal Biases in AI
Machine learning systems are trained on large datasets, which often reflect existing societal patterns and inequalities. Consequently, AI can replicate these biases or even amplify them in its outputs. For example, if trained on biased data, AI might provide more accurate diagnoses for groups well-represented in the data while producing errors or suboptimal treatment recommendations for underrepresented groups.
A stark example comes from a study by the University of Leeds, which showed that up to half of women presenting symptoms after a heart attack receive an incorrect diagnosis because common protocols are more tailored to typical male symptoms. If AI suggests treatments based on biased data patterns, it risks perpetuating inequalities in care. When patients perceive that AI systems may not be fair or accurate for everyone—particularly minorities or vulnerable groups—trust in the technology and healthcare institutions can be seriously undermined.
Privacy Protection
Medical data, being highly sensitive, is protected by strict regulations to ensure its privacy and security, such as the GDPR in Europe and HIPAA in the U.S. These laws require that any collection, storage, or processing of health data complies with rigorous standards to prevent leaks and unauthorized access. The transfer of such data outside hospital environments poses additional risks, necessitating that external providers maintain protection levels equivalent to those required within hospitals. Even without direct access, the possibility of malicious third parties accessing sensitive information represents a significant privacy risk. Managing external data in AI systems is particularly complex and must involve advanced security measures and compliance protocols.
Federated Learning: Advantages and Limitations
Federated Learning (FL) offers a solution to these challenges through a decentralized approach. Instead of transferring data to a central server, FL allows models to be trained locally within each institution or device, without data leaving its origin. This approach safeguards patient privacy, as sensitive information never leaves its source. FL also facilitates collaboration among hospitals and healthcare institutions by aggregating training results from different entities without compromising privacy.
This collaborative approach improves model representativeness and accuracy by integrating information from diverse populations and clinical contexts, reducing biases stemming from limited or single-source datasets. FL enables the creation of robust, inclusive models applicable to a broader patient demographic.
The Role of Trusted Execution Environments (TEEs)
Although FL minimizes data privacy risks, it still faces challenges related to confidentiality and computation integrity, which heavily rely on trust among involved parties. Trusted Execution Environments (TEEs) provide critical advantages in FL by creating secure zones on devices where sensitive code and data can be processed without exposure. TEEs ensure privacy and computation integrity and can issue cryptographic certificates to demonstrate data authenticity and integrity. These features make TEEs essential for adopting FL in highly regulated environments, ensuring secure and reliable data handling during distributed training.
A European Alliance for Efficient and Personalized Healthcare
The FLUTE project, funded by the European Commission, aims to develop an AI algorithm for diagnosing clinically significant prostate cancer (csPCa) through a federated and secure platform. Hospitals from Spain, Belgium, and Italy will participate in a pilot study in 2025 to evaluate the solution’s effectiveness in a real multinational setting.
The algorithm seeks to improve prostate cancer detection by identifying its aggressiveness while avoiding unnecessary biopsies. This approach is designed to enhance patient well-being and significantly reduce associated costs. At Gradiant, over 10 experts are working on a fused AI model that integrates clinical variables, MRI imaging, and biomarkers for diagnosis while managing model federation. Additionally, we are developing and integrating TEEs to secure hospital server hardware where the models will be deployed.
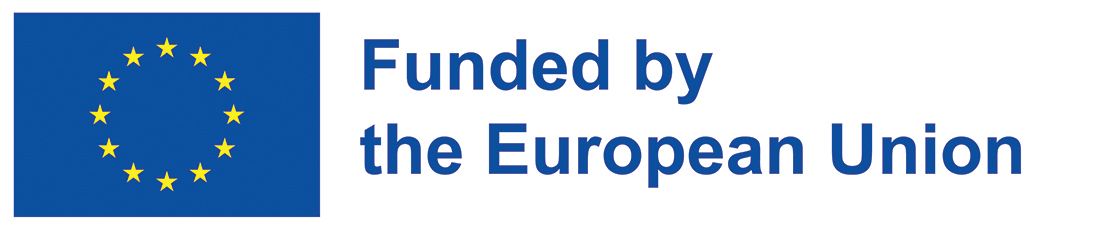
FLUTE project has received funding from the Horizon Europe Framework Programme (HORIZON) Research and Innovation Actions under grant agreement Nº 101095382.